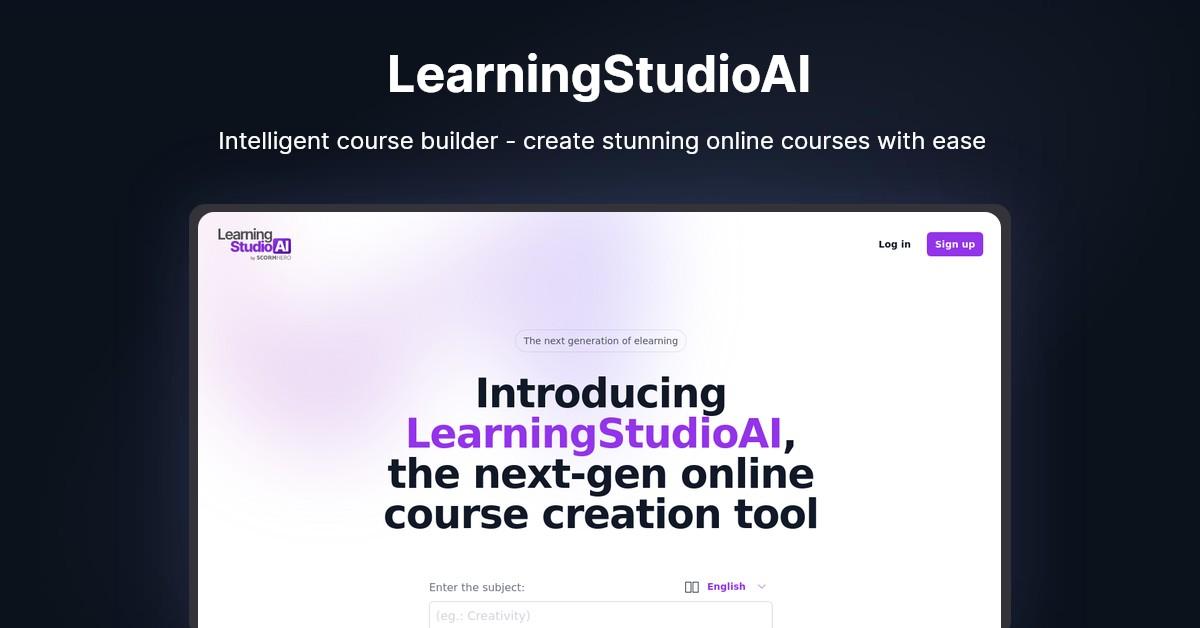
Introduction to Generative AI
Introduction to Generative AI and its applications
Generative AI refers to artificial intelligence techniques that generate new information, such as images, text, or audio, based on input data. These systems can create realistic content, leading to applications in various fields like art generation, content creation, and even drug discovery. By utilizing algorithms such as GANs (Generative Adversarial Networks) and VAEs (Variational Autoencoders), generative AI has the potential to revolutionize how we produce and interact with digital content.
Importance of Generative AI in various industries
Generative AI holds significant importance across industries like healthcare, entertainment, and marketing. In healthcare, it can assist in medical imaging analysis and drug discovery. In entertainment, it can aid in creating realistic CGI for movies and games. Furthermore, in marketing, generative AI can personalize content and improve customer engagement. The versatility of generative AI makes it a valuable tool for innovation and problem-solving in numerous sectors.
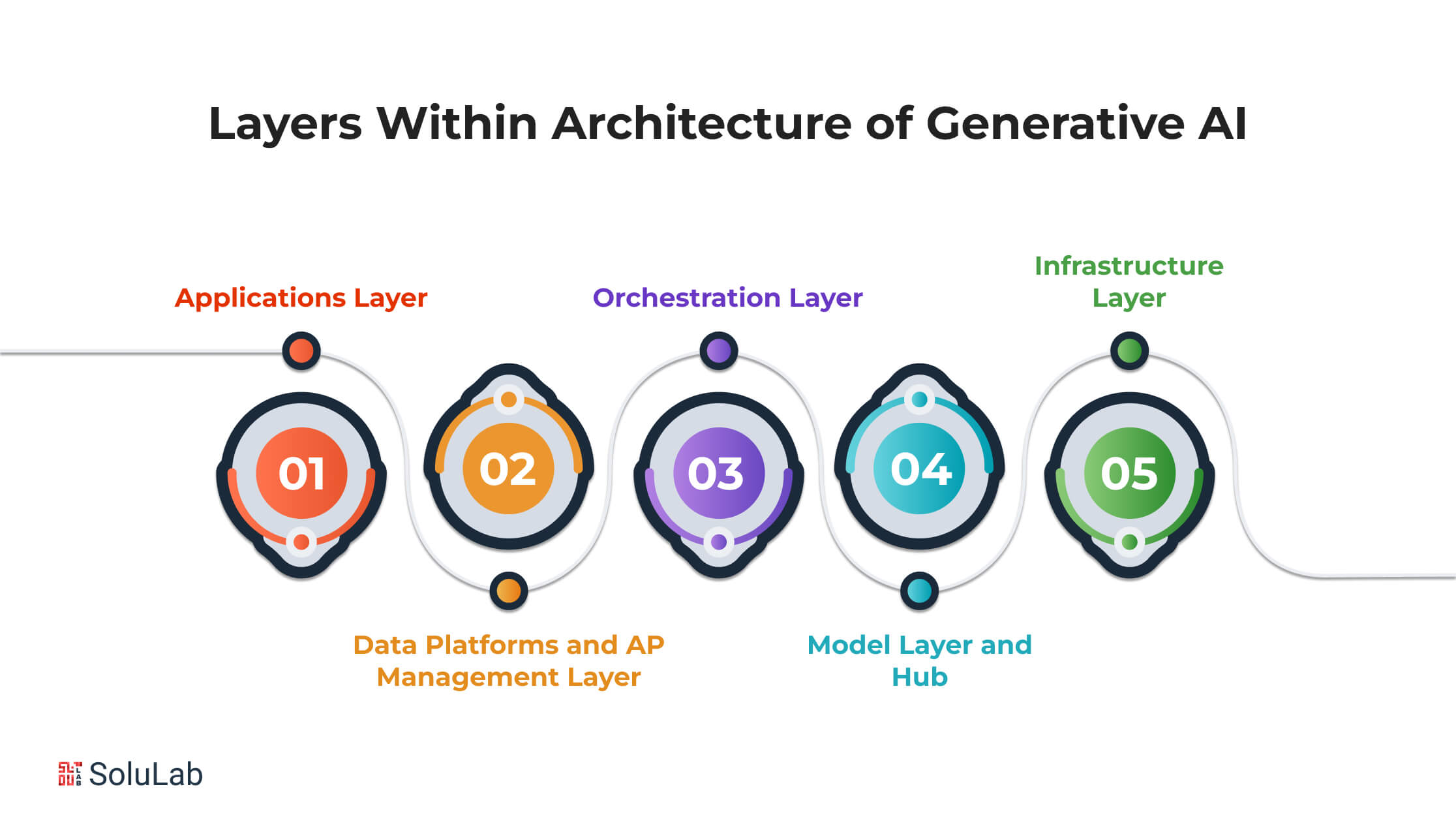
Understanding Neural Networks
Neural Networks basics and architecture
Neural Networks serve as the backbone of generative AI technology, leveraging interconnected nodes to mimic the human brain's functionality. Through layers of neurons, neural networks process and analyze data to generate outputs. This architecture allows for complex pattern recognition and information synthesis, enabling the creation of realistic content in various forms, enhancing innovation across industries.
Deep Dive into Neural Networks for Generative AI
Delving deeper, the specialized Neural Networks utilized for generative AI, such as GANs and VAEs, enable data generation based on learned patterns. GANs facilitate the competition between generator and discriminator networks to refine output authenticity, while VAEs focus on encoding data into meaningful representations for diverse applications, showcasing the breadth of generative AI's capabilities.
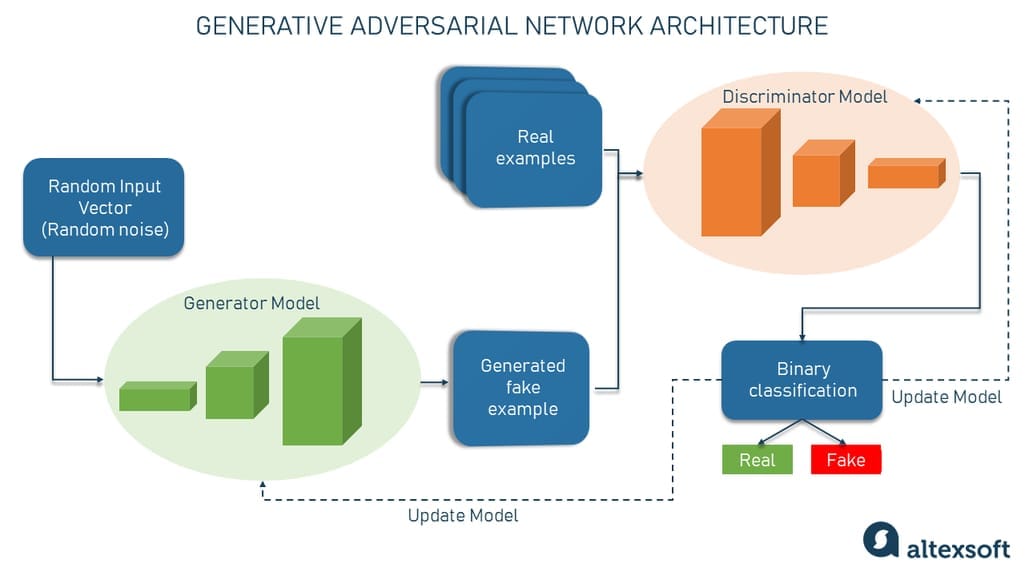
Training Generative Models
Process of Training Generative Models
Neural Networks play a crucial role in the realm of generative AI, functioning akin to the human brain to process and analyze data for innovative content creation. Specialized networks like GANs and VAEs refine data generation through intricate competition and encoding mechanisms. GANs enhance authenticity by pitting generator and discriminator networks against each other, while VAEs focus on creating meaningful data representations for diverse applications. These models showcase the depth of generative AI's capabilities.
Optimizing Training for Generative AI
Delving into training generative models, optimizing the process is essential for achieving desired outcomes. By fine-tuning parameters, adjusting data inputs, and refining network architectures, the training phase ensures the generation of high-quality outputs. Through meticulous optimization, generative AI models can enhance their efficiency and produce more accurate and innovative results across various industries.
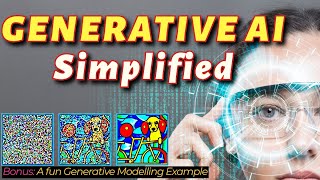
Generative Adversarial Networks (GANs)
Explanation of Generative Adversarial Networks
When delving into advanced generative AI techniques, Generative Adversarial Networks (GANs) stand out as pivotal models. GANs operate through a unique setup involving a generator network that produces synthetic data and a discriminator network that evaluates its authenticity. The adversarial nature of GANs drives the generator to continually refine its outputs to deceive the discriminator, resulting in the generation of highly realistic and novel data.
Applications and impact of GANs in Generative AI
The applications of GANs in the realm of Generative AI span various industries, including art generation, image manipulation, and data augmentation. By leveraging GANs, businesses can enhance their creative processes, develop realistic simulations, and even improve security measures through anomaly detection. The impact of GANs on Generative AI is profound, revolutionizing how data is synthesized and opening doors to unprecedented innovations in artificial intelligence.
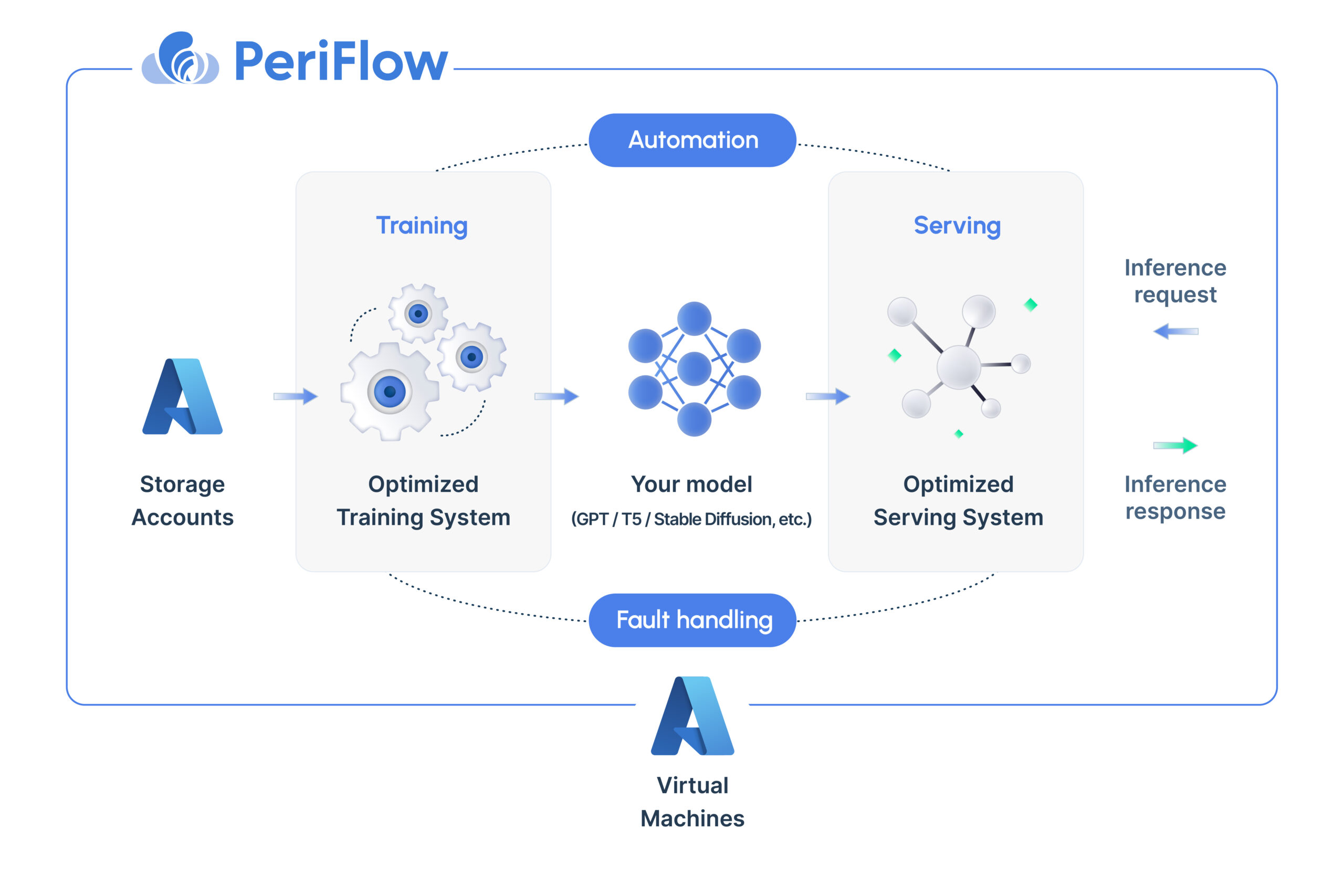
Conditional Generative Models
Concept of Conditional Generative Models
In the realm of advanced generative AI techniques, Conditional Generative Models play a crucial role. These models function by generating data under specific conditions or constraints, allowing for more targeted and tailored outputs. By incorporating additional information or context into the generation process, Conditional Generative Models can produce highly customized and relevant synthetic data, making them valuable tools in various applications.
Examples and use cases of Conditional Generative Models
The applications of Conditional Generative Models are diverse and impactful, spanning industries such as personalized content generation, image-to-image translation, and interactive storytelling. These models are utilized in creating bespoke recommendations, generating tailored designs, and facilitating dynamic content creation based on user input. Through their versatility and adaptability, Conditional Generative Models contribute significantly to enhancing user experiences and driving innovation in artificial intelligence.
Comments
Post a Comment